
This is all pretty easy to express in code: That is, we're looking to minimize the maximum distance from the targets, and maximize the mininum distance from the bad words. (When the target distances are smaller, it means the candidate is better.) That is, for each word $w$ in our dictionary we want to compute: One way to do this is to calculate, for a given candidate clue, the sum of its distances from the bad words minus the sum of its distances from the target words.

We can express the Codenames problem as taking a set of "target" words and a set of "bad" words, then trying to find candidate words that are close to the targets and far from the bad words. All this seems difficult for a computer to do.īut if we recast the problem in terms of our vector space model, where distance is a measure of semantic similarity, then finding a good Codenames clue becomes about finding a word that is close to the target words while being far away from all the others. You connect "GRENADE" to "PALM" because you know that grenades are held in your hand when you think of the two words together, you might even mentally simulate a throw. You connect "NARWHAL" to "NET" because you know that narwhals might be caught in nets. Codenames as an AI problem ¶Ĭodenames seems like a good Turing test: to come up with a clue, you need to not only understand the many shades of meaning each word can take on-"PAN," for instance, can be a piece of kitchenware, a way of criticizing, or a prefix meaning "all"-you also seem to need a model of the world. The GloVe vectors we'll be using were trained on 42 billion words worth of text gotten from the Common Crawl. (It uses a fancier method than the one described above.) It's just a list of words followed by 300 numbers, each number referring to a coordinate of that word's vector in a 300-dimensional space. Luckily, Stanford has published a data set of pre-trained vectors, the Global Vectors for Word Representation, or GloVe for short. It's a computationally intense procedure. After training across the entire corpus, the vectors come to embody the semantics latent in the patterns of word usage. At the heart of this neural network is a big matrix which has a column vector for each word in the training process, you're esssentially nudging these vectors around. Your goal is to predict the target from the context: you rejigger the weights of the network such that, based on the nine context words, it assigns a high probability to the tenth. Then, you read the text into a small moving window, considering maybe ten words at a time-nine "context" words and one target word.
MYSTIC WORDS APP ANSWERS DOWNLOAD
One way to generate word vectors uses a neural network: you download a vast corpus of text, say all of Wikipedia. "Word vectors" attempt to quantify meaning by plotting words in a high-dimensional space words that are semantically related end up close to each other in the space. It can be delightful, and frustrating, to see your friends' minds leap from idea to idea-often going places you never intended.Ĭan you think of a clue for the board above? The game is interesting because it requires you to connect far-flung concepts precisely enough that other people can re-create your associations. (There are rules about which kinds of clues are allowable: usually it has to be a single word proper nouns are optionally allowed.) Your task is to come up with a single word that connects HAM, BEIJING, and IRON, while avoiding the others. The tan words are neutral or perhaps belong to your opponent.
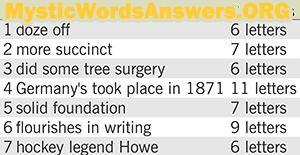
The black word is the bomb if your teammates say that one, they instantly lose the game. The three blue words are the target words-that's what you want your teammates to guess. The real game is played on a 5x5 board, but here is a typical situation faced by a clue-giver:
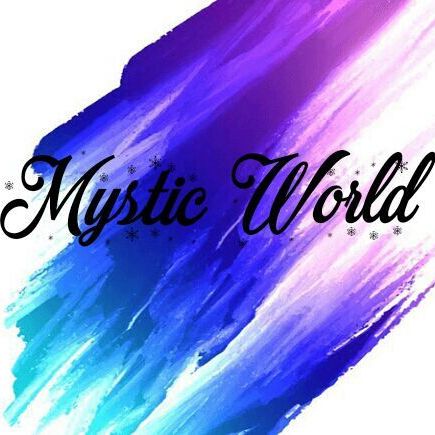
What is Codenames? ¶Ĭodenames is a Czech board game by Vlaada Chvátil where the goal is to say a one-word clue to your teammates in order to get them to choose correctly from the words laid out on the table. Automatically finding Codenames clues with GloVe vectors ¶Ībstract: A simple vector-space model shows a surprising talent for cluing in the Codenames board game.
